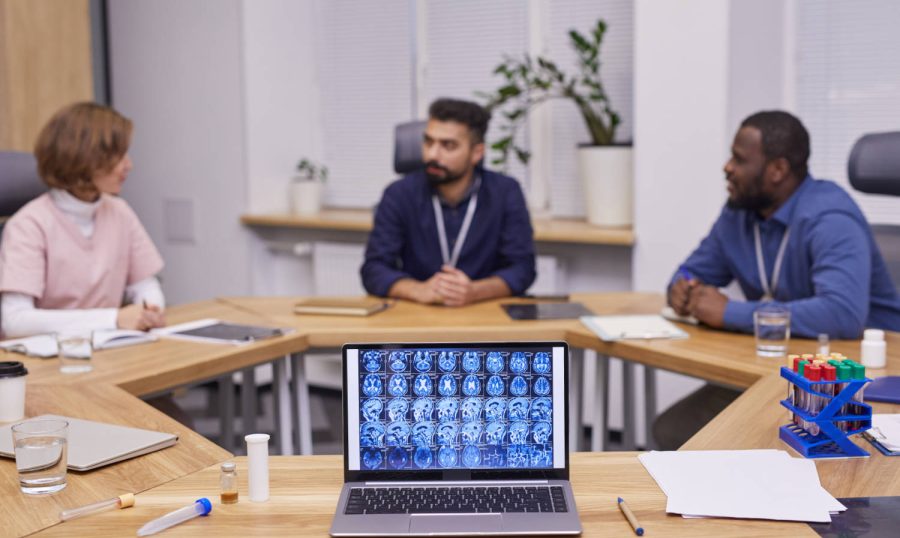
The application of Artificial Intelligence (AI) in the analysis of brain scans represents a transformative leap in the field of neuroscience, enabling more accurate and timely diagnosis of neurological disorders. Traditionally, brain scans, such as magnetic resonance imaging (MRI) and positron emission tomography (PET), require expert interpretation, which can be time-consuming and prone to human error. AI algorithms, particularly deep learning models, are being developed to automatically analyze these scans and identify subtle patterns that are indicative of conditions such as Alzheimer’s disease, Parkinson’s disease, multiple sclerosis, and even brain tumors. These advancements are revolutionizing the diagnostic process by allowing for earlier detection, which is crucial for improving patient outcomes.
AI models, particularly convolutional neural networks (CNNs), have shown significant promise in detecting biomarkers for neurological disorders that might not be easily visible to the human eye. For example, in Alzheimer’s disease, AI algorithms can identify early changes in brain structure, such as atrophy in specific regions like the hippocampus, which may not be immediately apparent in conventional clinical assessments. By analyzing vast amounts of brain imaging data, these AI systems are trained to recognize patterns associated with various stages of neurological diseases, enabling more precise monitoring of disease progression. Furthermore, AI’s ability to process and integrate large-scale data from multiple brain scans enhances its ability to make predictions about disease onset and progression with unprecedented accuracy.
In addition to diagnosis, AI is being explored for its potential in personalizing treatment plans for patients with neurological disorders. By analyzing brain scans alongside genetic, clinical, and behavioral data, AI models can offer insights into which treatments might be most effective for individual patients. For example, in the case of brain tumors, AI can assist in planning personalized treatment strategies by analyzing tumor location, size, and its relationship with surrounding brain structures, optimizing the approach for surgery or radiation therapy. AI is also being used to monitor the effectiveness of treatments in real time, providing clinicians with continuous feedback and enabling adjustments to therapy as needed.
Despite the impressive capabilities of AI in brain scan analysis, challenges remain, particularly around data quality, interpretability, and ethical considerations. Brain scans can vary significantly due to factors like imaging technology differences, patient movement, and age-related changes, which may affect the reliability of AI predictions. Furthermore, the “black-box” nature of some AI models raises concerns about transparency and trust in clinical settings. Ensuring that these AI systems are interpretable and can provide clear rationales for their predictions is essential for their widespread adoption in healthcare. Additionally, the integration of AI into clinical workflows must be done carefully to avoid potential biases and ensure equitable access to advanced diagnostic tools. As research progresses, AI’s ability to assist in the analysis of brain scans will likely become a cornerstone of precision medicine, leading to earlier interventions, more personalized treatment options, and improved outcomes for patients with neurological disorders.