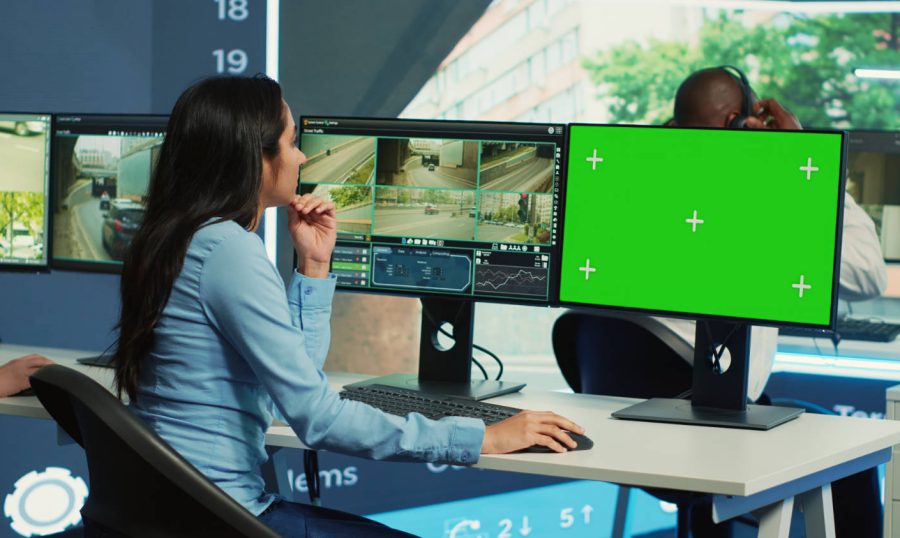
Computer Vision is a rapidly evolving field within artificial intelligence (AI) that focuses on enabling machines to interpret and understand visual information from the world, much like humans do. By processing and analyzing digital images and videos, computer vision systems are designed to extract meaningful insights, make decisions, and perform tasks that typically require human visual perception. The goal is to equip machines with the ability to recognize objects, understand scenes, detect anomalies, and track movements, allowing them to interact with the environment in a more intuitive way.
Key techniques in computer vision include image classification, object detection, semantic segmentation, and image generation, each serving different applications across industries. For example, image classification involves categorizing images into predefined classes (such as identifying whether an image contains a cat or a dog), while object detection not only identifies objects but also locates them within the image. Semantic segmentation goes a step further by labeling each pixel in an image, which is particularly useful in tasks like medical image analysis. With the rise of deep learning and convolutional neural networks (CNNs), computer vision has seen significant breakthroughs, enabling high accuracy in tasks such as facial recognition, self-driving cars, and visual search engines.
The development of computer vision has been powered by the availability of large-scale datasets and computational resources. Data-driven approaches, particularly deep learning, allow models to learn directly from raw image data without needing manual feature extraction. These models are trained on vast amounts of labeled data, making them highly effective in handling complex visual tasks. For instance, in autonomous vehicles, computer vision enables real-time object detection and scene interpretation, allowing cars to navigate safely. Additionally, computer vision is making strides in healthcare, such as aiding in early disease detection through the analysis of medical imaging like X-rays and MRIs.
Despite the progress, challenges remain in the field of computer vision. Variability in lighting, angles, and occlusion can make it difficult for machines to accurately interpret images in real-world scenarios. Moreover, issues related to bias in training data, ethical concerns around surveillance, and privacy implications are also critical areas of focus. As the field continues to advance, interdisciplinary efforts are being made to improve the robustness, fairness, and generalization of computer vision systems, ensuring that these technologies benefit society while minimizing potential risks. The future of computer vision holds immense promise, with applications ranging from augmented reality and retail to healthcare and security, transforming how machines perceive and interact with the visual world.